All gain, no pain: How AI and machine learning elevate ad creative with no extra work
By John Tigg, GM, International, Yieldmo
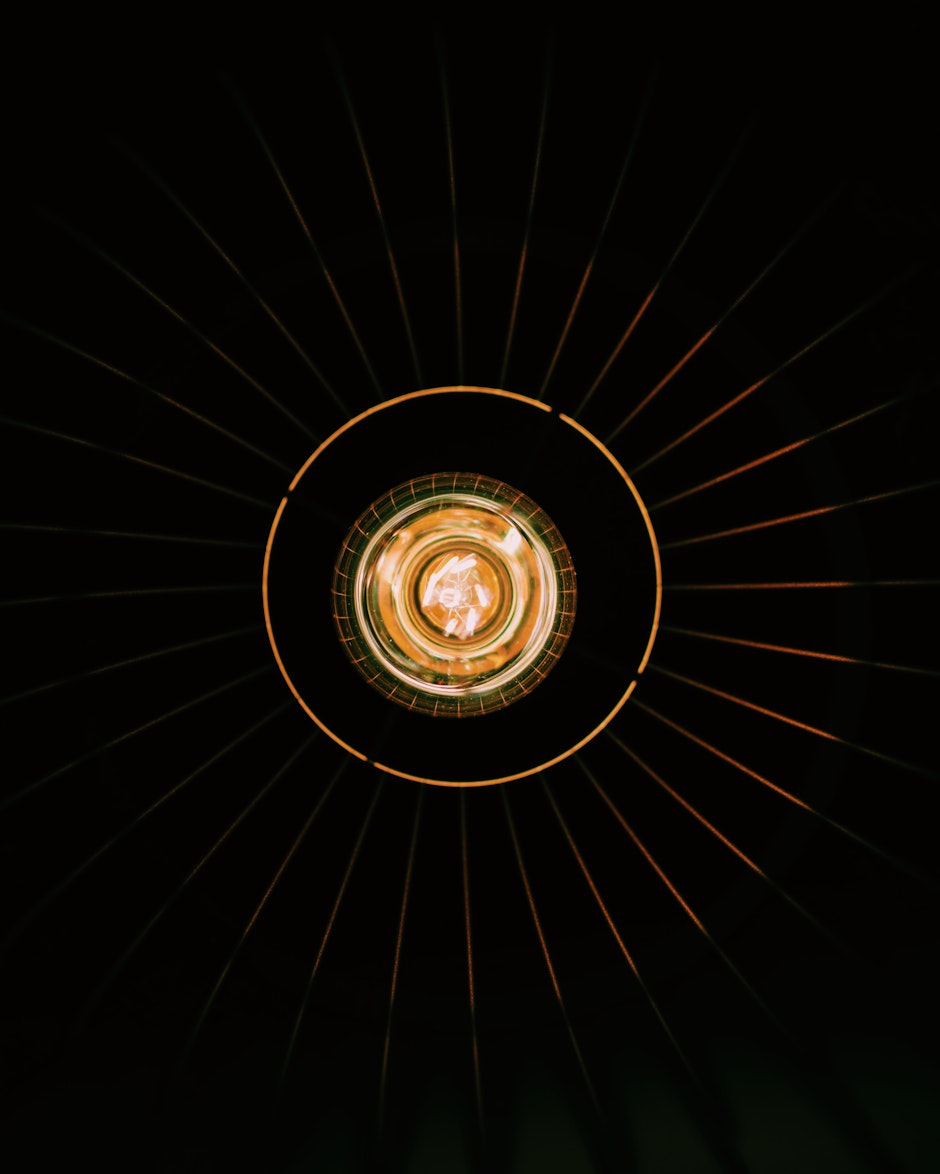
Claims of a sentient Google chatbot, and the surreal images generated from text prompts by the DALL-E drawing tool, have the media, public and policymakers captivated by the dream (or the nightmare) of artificial intelligence (AI). On the other hand, there are those of us who have been working in the field of AI for decades who have seen this hype cycle play out before, now backed by researchers who are urging people to reset their expectations.
As it is currently used, AI — or, more specifically, this subset of machine learning — is a powerful tool for sorting and deciphering vast and complex volumes of data. The more data a machine learning model is fed, the better it gets at recognizing useful connections. In the data-heavy digital advertising world, data is not just information, it is currency, and the more value marketers and publishers can extract from it, the more they can profit from buying and selling inventory.
The industry has invested heavily in AI, and it has paid off, with 79% of global marketing and sales professionals reporting an increase in revenue after its adoption. Machine learning models have been taught how to optimize ad content and placement, recognize contextual clues, and match the right inventory with campaign KPIs. While these algorithms may not have any original thoughts, they are very good at being told what to do.
Machine learning serves the right ad in the right place to maximize impact
Marketers can tailor their content to individual consumers using AI, and there are two machine learning models that can be used in tandem to achieve this:
Dynamic creative optimization (DCO)
DCO harnesses data sources to match the message of an ad to the context of the inventory. It takes in signals such as location, content on a web page, and how the device is being used to rapidly change messaging to reflect the consumer situation. For example, a holiday company can change its messaging depending on where the viewer is based, what time of year it is, and what device they are viewing the ad on.
Marrying machine learning with programmatic buying means that these changes can be made in-flight with the most effective messaging being delivered rapidly to a consumer.
Dynamic format optimization (DFO)
DFO works similarly to DCO, except instead of customizing the content of an ad it customizes the ad placement. For example, the machine learning model can present the creative in a different format or different location on the screen depending on which is most likely to make an impact depending on various contextual clues.
Like DCO, all calculations are performed in real-time, again responding to the signals of each unique consumer and optimizing the campaign while it is in-flight.
Programmatic has been powered up by machine learning
Machine learning forms the core of both solutions, and for true optimization, these technologies need to be present throughout the ad buying process.
AI can provide value from the moment a request comes into an ad exchange. Programmatically-run ad campaigns are created with a KPI in mind — whether it be impressions, click-throughs, or plays — then predictive machine learning models facilitate a deeper understanding of how these goals can be attained and predict a range of outcomes.
The machine learning models that can power these ad exchanges are fed vast quantities of signals relating to ads, everything from more traditional clicks and views to phone tilts and scroll speed. When an ad request is made on an exchange, machine learning models rapidly analyse the request, consider the campaign's goals, and then search for inventory that best maximizes the campaign potential.
This is possible thanks to the ‘learning’ aspect of AI. The models can process billions of combinations of data points, spotting trends and patterns that are related to specific outcomes, then searching for the inventory that will help advertisers make the best return on their ad investment. DFO takes this a step further, using these models to find not only the best place but the best format for an ad to appear in.
AI can help fill the cookie-shaped hole in the industry
Marketers are currently stuck between a rock and a hard place. On the one hand, a global recession looms, and consumer confidence is reaching record lows. On the other, the gradual retreat of third-party cookies from the data menu has the industry scrambling for privacy-compliant alternatives.
Machine learning and AI models can help brands walk this tightrope, delivering increased ROI while targeting in a privacy-secure fashion. The key to this is the ability of these models to be built around non-addressable and contextual signals, as seen in Encantos’ use of Yieldmo’s Contextual Curation Engine, which resulted in a 48% increase in CTR and 72% increase in viewability compared to non-optimized, non-addressable impressions.
This method of targeting is also platform agnostic, allowing advertisers to optimize on the open web in ways usually only available in increasingly powerful walled gardens. While these environments can be fruitful for reaching consumers, they may not always be the contextually correct landscape and user purchase intent could be highest elsewhere. When using social media sites, for example, it is likely that users are not looking to shop, but instead, connect with friends.
Right now, AI is a long way from being able to trap us all in The Matrix, but it is a powerful tool to help advertisers supercharge their creatives. The ability of machine learning models to maximize ad potential using privacy secure signals, before tailoring ad experiences to the user on a page using contextual signals, means AI adoption will decide which brands thrive as third-party data depreciates.